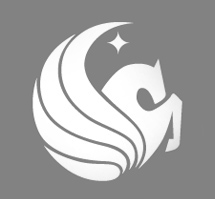
Eshed Ohn-Bar
Max Planck Institute for Intelligent Systems
Monday, March 11, 2019
3:30PM – 4:30PM – HEC 438
Abstract
How can we design data-driven, computational algorithms that effectively reason over the behavior of humans in real-world, safety-critical, shared autonomy scenarios? This is the main motivating question for my research. Computational frameworks for perception, prediction, interaction, and collaboration with humans are key to realizing ubiquitous autonomous and assistive technologies, e.g., self-driving vehicles that can interact with humans safely, and assistive systems for guiding a person with visual impairments in unfamiliar, complex environments. However, there are still several fundamental challenges in modern machine perception and learning approaches that must be addressed before ubiquitous human-interactive autonomous and assistive technologies can be realized.
In this talk, I will focus on raising and addressing two issues in machine perception and action for embodied, real-world systems. By addressing the two issues, I will show how we can achieve a more effective integration between system perception and action in human-interactive environments. First, robust perception and modeling of humans at varying levels of abstraction (e.g., object, scene, activity, and intent-level) is difficult and cannot be assumed even with state-of-the-art approaches. To address this issue in machine perception, I will develop a more human-like, attention-based perception framework that enables learning functional and contextual perception models. The proposed framework leverages human guidance during training to learn a notion of situational awareness, while also mitigating dataset bias and generalization issues. I will demonstrate the learned models to be particularly suitable for safety-critical tasks, such as mobility and human interaction. Second, even once a robust model has been obtained, human-interactive systems must operate alongside dynamic and highly diverse human behavior, users, and environments. To tackle this second issue in machine action, I will present a data-efficient interactive learning framework for assistive systems, and study it in the context of assistive navigation of a blind person. The general learning framework is specifically suited for collaboration with diverse users and environments. Based on real-world experimental analysis, I will show how the proposed learning framework efficiently adapts to diverse blind users while enabling long-term prediction of user behavior. The two frameworks provide a step towards perception-action robotic systems that operate with and alongside humans.
Biography
Eshed Ohn-Bar is a Humboldt research fellow at the Max Planck Institute for Intelligent Systems. Previously, he was a post-doc at the Computer Vision Group and Cognitive Assistance Lab in the Robotics Institute at CMU. His work has been awarded the 2017 best PhD dissertation award from the IEEE Intelligent Transportation Systems Society, a double best student paper award honorable mention at ICPR 2016, the best industry related paper award honorable mention at ICPR 2014, and the best paper award at the IEEE Workshop on Analysis and Modeling of Faces and Gestures at CVPR 2013. He co-organized several workshops on machine perception and learning for intelligent vehicles at CVPR, ICCV, and IV conferences. He is also an Associate Editor for the IEEE Intelligent Vehicles Symposium 2019. Eshed received the BS degree in mathematics from UCLA in 2010, MEd from UCLA in 2011, and the PhD degree in electrical engineering from UCSD in 2017.